How machine learning could help Simone to play Ludo
By Helga Haberfehlner
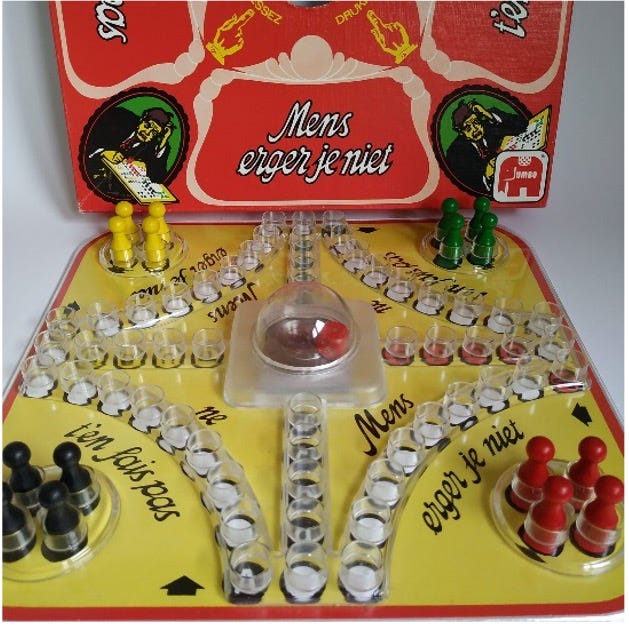
Simone is a 12 year old girl. She loves to play Ludo with her mum, dad and her little brother. However, when she pushes the dice switch in the middle of the Ludo board game, she cannot properly control her movements and the tokens sometimes fall from the board. Simone also does not succeed to move the tokens, so her dad does that for her. Simone has dyskinetic cerebral palsy, due to a lack of oxygen around birth. Involuntary movements (called dystonia and choreo-athetosis) disturb her during daily activities such as playing Ludo.
At Amsterdam UMC, we treat children with severe dyskinetic cerebral palsy with medication that is directly delivered into the spinal canal by an indwelling pump (intrathecal baclofen). This treatment has been shown to be effective in reaching individual goals (such as not overthrowing the tokens when playing Ludo). However, we currently have a hard time to exactly monitor the involuntary movements and the effect of treatments on them. This monitoring is very important to give the right doses of medication. During check-ups at the hospital, doctors ask parents and children, how movements evolve and observe the children in the consultation room. However, in this way, we are only able to capture a snapshot within the hospital environment of the involuntary movements. Therefore we are seeking for options to measure movements within the natural environment of the children (such as home and school), and to automatize the evaluation to make it not too time consuming for the doctors.
Activity recognition applications using sensors integrated in smartphones and smartwatches for sports and fitness are increasingly used and could possibly serve this purpose. Machine learning models integrated in the devices can distinguish between movements such as walking, jogging, climbing, running and swimming. Hence, such sensors might be a good candidate to detect and monitor the involuntary movements of Simone while playing Ludo. Furthermore, as also information extracted from videos could be useful, parents could easily film children at home with their smartphones, to obtain additional information.
We have recently started to explore the possibilities for automatic detection of involuntary movements in children with dyskinetic cerebral palsy in the home situation. ZonMw financed our project MODYS@home to use smartwatch-like sensors at home for the assessment of involuntary movements. With this support, we could develop an app to measure sensor data together with synchronized videos during daily activities.
We also received help from the Netherlands eScience Center within the ‘Open Call for Small-Scale Initiatives in Machine Learning’. Within this project called ‘Automated video-based movement assessment using machine learning to support personalized treatment of movement disorders’, we focus on the option to use videos. We use stick figure movies extracted from real videos.

Real videos were selected from other projects, that had have been scored by the doctor for involuntary movements (dystonia) for the arms and legs. With these data we trained machine learning models to predict dystonia automatically.
Using machine learning within the field of clinical movement analysis was something new for us, as not only the content was new, but also the style of working with stand-up meetings and sprints, sharing codes on Github and right away publishing our dataset. We gained new knowledge and skills that brings us a step forwards to find a way to measure children with dyskinetic cerebral palsy within their home environment.
We are very excited about the results from a random forest regressor, “a traditional machine learning model” as such a model is called in the language used in machine learning world ;-) See figure for the result: the true score by the doctor is plotted against the predicted value by the model. All scores on the diagonal line are predicted completely correct. The model is not perfect yet, but it shows the potential of the method and we are looking very much forward to further improve it.

Within our projects we work within a group of clinicians, researchers and software engineers from the Amsterdam UMC (Annemieke Buizer, Laura Bonouvrié, Marjolein van der Krogt, Helga Haberfehlner, Shankara van der Ven, Dylan den Hartog), from the TU Delft (Jaap Harlaar), from Moveshelf (Ignazio Aleo, Johannes Gijsbers) and the Netherlands eScience Center (Sven van der Burg, Florian Huber and Sonja Georgievska).
If you are interested in more technical details please have a look at our stick figure dataset. All contribution to our code is welcome, to help Simone to play Ludo without overthrowing the tokens!